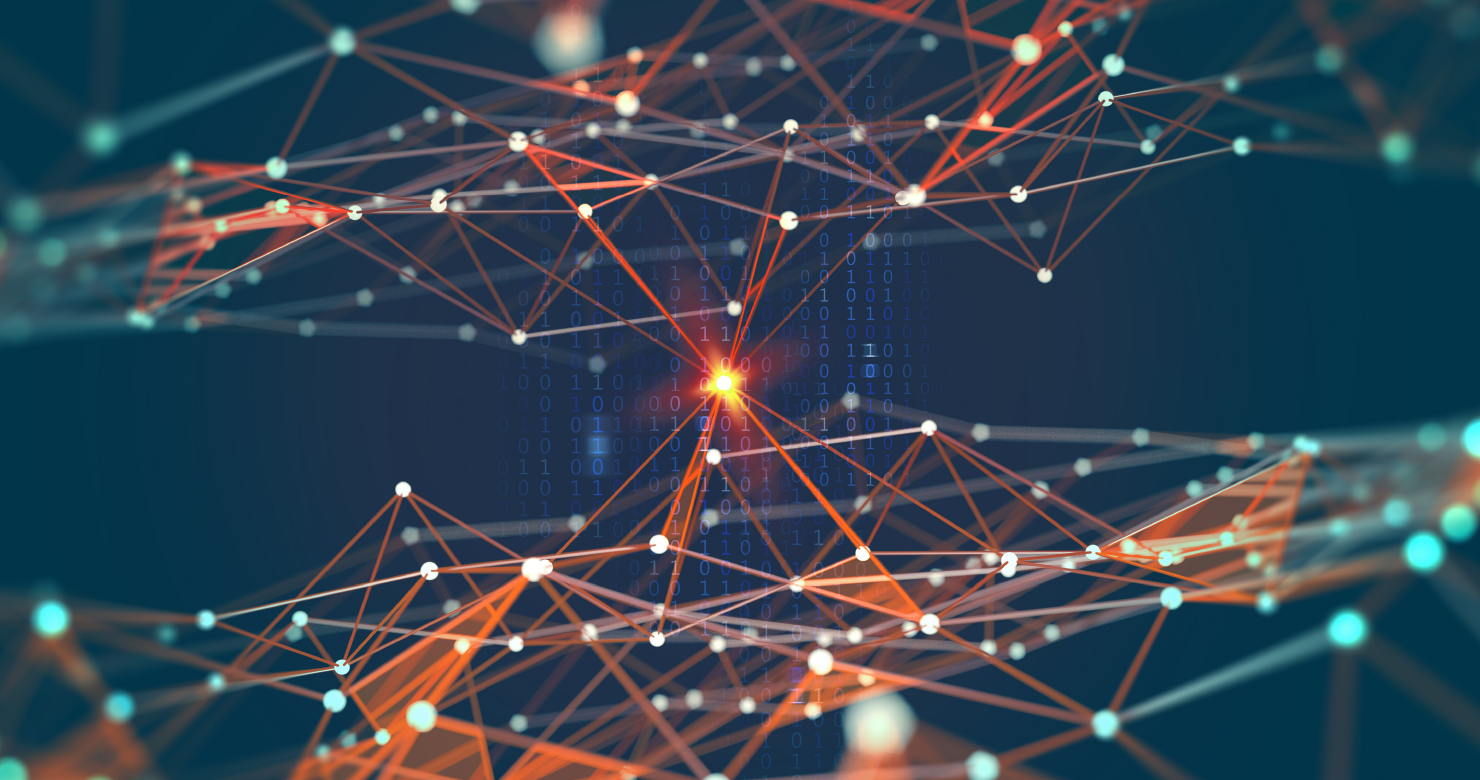
Machine vision technology is now widely used for surveillance, and researchers are working to make it more and more intelligent. There have been recent advancements in the detection of people, recognition, and tracking, but occlusion is still a well-known issue in people-detection.
Occlusion occurs when the person or object that is desired to be seen is partially covered by an intervening body or object. Occlusion is often a concern in crowded public areas, such as city squares. Incidentally, these areas are often locations where machine vision is highly-useful to help protect the public.
Challenges When Constructing Complete Images
Occlusion generally affects any machine vision technology. When an occlusion occurs for machine vision, that portion of the object is unknown, and to most intelligent surveillance systems, it does not exist if it cannot be observed. A system used to construct complete images must first know that an image is incomplete.
Another major challenge to advancement when it comes to occlusions is the lack of large-scale datasets that provide realistically occluded and non-occluded pairs of images. Simply adding random objects and textures to a non-occluded image fail to generate realistic data. Training a neural network with such data can actually be a liability to the system.
Another major challenge to advancement when it comes to occlusions is the lack of large-scale datasets that provide realistically occluded and non-occluded pairs of images. Simply adding random objects and textures to a non-occluded image fail to generate realistic data. Training a neural network with such data can actually be a liability to the system.
Machine Vision and AI Overcome Occlusion
Researchers are trying to add the human capability of “filling in the gaps” to machine vision systems. By using deep-learning architectures and photographs and video of people with and without occlusions, AI solutions can now figure out the missing information and create a complete image of a person.
Neural network architectures provide the framework needed to de-occlude shapes of people. The use of state-of-the-art U-nets (convolutional neural networks designed for fast and precise segmentation of biomedical images), GANs (generative adversarial networks used for unsupervised machine learning), and discriminative attribute classification nets (used for quality inspection) make de-occlusion possible.
An optimized loss function then uses the data in the aforementioned network technologies to meet the following objectives:
- -- Form an image without occlusion
- -- Have a similar pixel level to a completely visible “person shape”
- -- Conserve similar visual attributes of the original
- -- Machine vision technology advancements open up many doors within the surveillance industry for increased public safety by overcoming occlusion challenges.
Machine vision technology advancements open up many doors within the surveillance industry for increased public safety by overcoming occlusion challenges.